Improving self-driving cars by understanding the urban environments of people across the E.U.
We’ve mapped a wide range of unique obstacles that self-driving cars may face in six different E.U. capitals, namely Berlin, Vilnius, Latvia, Zagreb, Ljubljana, and Lisbon.
Task
Create awareness around the variety of local challenges image recogntition systems of self-driving cars can face in the E.U.
As we place self-driving cars (SDCs) in the real world, the cars will need to understand specific real-world environments. But what exactly do they need to understand? What are the obstacles they will encounter?
SDCs have been known to fail to recognize their surroundings in manners that baffle most humans. For instance, they failed to properly recognize kangaroos, traffic signs, and people with a dark skin. In some cases, such obscure failures are hard to foresee. However, in many cases, helping the data science teams behind the SDCs better understand the environments in which the cars will drive, can solve problems early in the development process, before resources are spent or damage is done.
Exploring six cities across the E.U., we see that each place has their unique obstacles. These can be grafitti culture in Berlin, to advertisements on in Zagreb, to road-side markets in Vilnius. Below, we describe two of our findings: Portugese pavement in Lisbon and advertisements in Zagreb. Feel free to contact us for the full report.
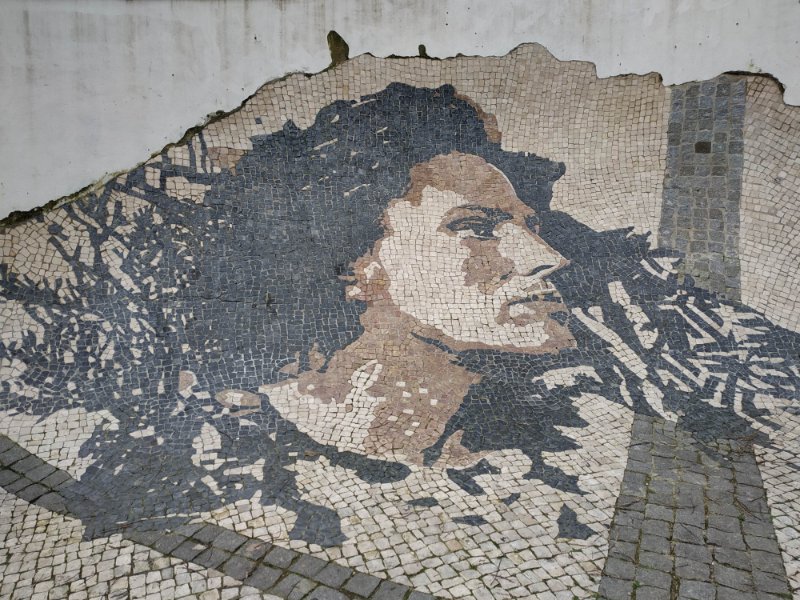
Calçade portuguesa – or Portuguese pavement – is a unique form of pavement found throughout Portugal. You recognize calçada portuguesa by their small pieces of flat stones laid in mosaic-like patterns. While beautiful and giving the city a lot of character, these pavements also cause difficulties for those walking on them.
As they are hard to walk on, calçade portuguesa also impacts the behavior and motion trajectories of pedestrians. Of course, a critical aspect of building self-driving cars (SDCs) is predicting exactly these pedestrian behavior and motion trajectories.
Issue #1: slipperiness
First, Portuguese pavement gets quite slippery when it rains. As people walk on them, the pavement wears down, resulting in very polished stones.
These polished stones, combined with wetness of the rain, form the ideal conditions to slip. In the picture above, you can see the surface glistening after a rain shower.
Issue #2: loose stones
Secondly, the pavement is also relatively hard to maintain. To make sure they are durable, the stones need to be laid by specialty pavers specifically trained to lay calçade portuguesa. Unfortunately, there are not a lot of these craftsmen available anymore.
Consequently, with the huge surface area covered by the pavements, it is almost impossible to keep them up to date. As a result, stones come loose and, once that happens, it’s easy for other stones to follow suit until inevitably a hole has formed.
Issue #3: hills and sidewalks
A third issue exacerbates the first two issues. Lisbon is a very hilly city, making slippery pavement and holes in them even more treacherous. What is more, because Lisbon is an old city, there are often a lot of roads with very narrow pavements because there’s no space for broader ones.
The above issues make walking in Lisbon an interesting experience. Guidebooks for first time visitors often tell people to prepare for the sidewalks and the steep hills by bringing fitting footwear such as sneakers. Many articles have been written about the danger of these pavements, including on how many people end up in the emergency room with injuries because of them.
Some Lisbon residents even have rules for walking on them, such as don’t walk on the pavement while drunk, don’t walk on the pavement and talk on your phone at the same time, and never pass a passerby on the right. Below, I present three pedestrian behaviors that result from people adapting to the unique qualities of calçade portuguesa.
1. Tripping, sliding, and falling
As you can imagine, slippery and loose stones in the pavement form a hazard to pedestrians. It is so easy to trip, slide, and fall. In fact, in a public survey on the pavements, 55% of respondents noted they had already fallen on the pavement, while 92% expressed a fear of falling on the pavement.
In my own conversations with residents, the experiences noted in the survey were confirmed. For instance, one resident told me how she slipped on the pavement because of the rain, while another told me about her experience of tripping while unexpectedly stepping into a hole in the pavement. In both cases, these residents had only been living in Lisbon for less than 6 months.
2. Walking on the street
Because of the danger of slipping, it is not uncommon for people – especially senior people – to walk on the road instead of the sidewalks when it rains. For instance, the lady in the picture below walked for a rather long time on av. Duque de Loulé, a busy street where cars drive particularly fast.
Of course, pedestrians walking on busy roads where sidewalks are available present unique challenges to drivers. For one, drivers have to pay extra attention in order to swerve around these pedestrians in time, as can be seen in the picture below.
To exacerbate the problem, darkness and rain make pedestrians less visible (people casually walking usually don’t have high-visibility apparel like, say, joggers do). Furthermore, the expectation that pedestrians normally walk on the sidewalks, makes it even harder to prepare for them being on the street.
3. Kicking loose stones
Finally – though likely less critical to drivers – people often kick loose stones laying on the sidewalks, either on purpose or by accident.
For instance, during my observations, one kid in a family of four started kicking a loose stone along while walking on the sidewalk. While his parents eventually told him to stop, stones can become a unpredictable projectiles. This was the case in another instance, where a loose stone hit an elderly man, though without much consequences.
Though these problems with the pavement exist and are widely known, simply replacing them with other types of pavement isn’t a solution. Portuguese pavements form a part of Portuguese Heritage, and many people find them critical to the identity of Lisbon. What is more, these pavements contribute much more to the permeability of the soil, as rain can seep in between the stones.
The tension between the simultaneous beauty and danger of the pavements is, we feel, nowhere as clear as in the Olivais subway station on the red subway line (see picture below). This station’s platform is paved with Portuguese pavement, but the pavement is covered by a smooth, transparent layer to prevent tripping, slipping and falling onto the rails.
The impact of calçade portuguesa might be easily missed when developing and testing self-driving cars. Holes in the pavement are certainly not everywhere, rain is rather rare in Lisbon during some seasons, and narrow sidewalks can’t be found everywhere. Direct observations of the above described behaviors are therefore rather unlikely, unless one looks for them.
What is more, pedestrian behaviors resulting in actual traffic accidents is often severely under-reported. This lack of official reporting, along with a lack of direct observations, might lead data scientists to not pick up on the issue.
Of course, the behaviors and their consequences are real. As such, it’s important to consider what impact the above issues and their resulting pedestrian behaviors mean for self-driving car systems.
1. Train models to distinguish between calçade portuguesa and other pavements.
State of the art semantic segmentation models trained on city images, such as Mask2Former, do not yet (understandably so) detect calçade portuguesa specifically. Nevertheless – considering the unique pedestrian behaviors described above – simply recognizing calçade portuguesa could already signal SDCs to drive more cautiously and to respond faster in dangerous scenario’s.
2. Expect pedestrians to walk on the road in unexpected places, especially when it rains.
Under normal circumstances, you would expect pedestrians in Lisbon to walk on the sidewalks. However, slippery and narrow sidewalks encourages some to walk on the road instead. Make sure that during rainy weather and in places with narrow sidewalks, the SDCs take extra precautions to detect pedestrians and correctly calculate their motion trajectories.
3. Understand there’s a higher chance of pedestrians tripping, slipping and falling.
Though pedestrians tripping, slipping and falling can always happen, there seems to be a higher likelihood of this happening in Lisbon. Prepare SDCs to understand these behaviors and adapt accordingly. Another option would be to detect holes in the pavement to detect high-risk areas early.
19 tram lines running through Zagreb connect different parts of the city. In most cases, the trams display advertisements on their sides. The visual content of these advertisements vary from simple words to pictures of babies with tennis rackets.
Similar advertisements can be found on walls very close to the road. Unfortunately, the model we tested (MSeg1080_RVC segmentation) failed to understand that the content of these advertisements was not real.
How the model sees a tram in Zagreb and mistakes advertisements for a fence (slide left/right).
In the above image, the tram’s advertisement covers and obscures most of the tram. Only the front part of the tram is recognized (as train and bus), while most of the remaining parts are recognized as “fence”. Similar faulty predictions on tram advertisements can be seen in the images below.
Faulty predictions (e.g. person, suitcase, etc.) of the model made on trams with advertisements (click to enlarge)
However, the model doesn’t always make faulty predictions on trams, as seen in the following two images (if train or bus is considered the correct label for tram). In the first image, a tram with pictures of people is recognized simply as a train, as it should be. In the second image, the tram displays no advertisements, which is much more easily recognized by the model.
Correct predictions of the model made on trams with and without advertisements (click to enlarge)
Billboards can be found all through Zagreb’s city center. At times, these billboards are placed on a wall almost right next to the road. They portray a wide variety of scenes, often with people and cars. Similar to the advertisements on trams as discussed above, the model often fails to distinguish between reality and fiction here as well.
How the model sees a billboard in Zagreb and fails to make a distinction between a picture of a car and a real car (slide left/right).
In the above image, a billboard displaying an advertisement of a car hangs close to the road. However, the model labels the image of the car as a car in itself. This difference between reality and fiction would have easily been picked up on by a human (i.e. seeing the border of the billboard or the text surrounding the car). Similar faulty predictions on billboards can be seen in the images below.
Faulty predictions (e.g. person, couch, etc.) of the model made on billboards (click to enlarge).
Of course, the images above are taken close to the billboards. This makes it understandably harder to distinguish between reality and fiction, even for humans.
When the images contain the surroundings of the billboards, the model has a much easier time labeling them as a billboard instead of its content. This can be seen in the images below. Nevertheless, in many cases in reality, billboards are placed close to roads which would obscure the surroundings.
Correct predictions of the model made on the billboard with a car on it (click to enlarge)
Overall, the MSeg1080_RVC model performs very well. Buildings, people, vegetation, cars, etc. were easily detected by the model in the current analysis. The accuracy and robustness of the model are truly impressive.
Nevertheless, several scenario’s from Zagreb resulted in faulty predictions which could lead to dangerous situations in real-world contexts. To prevent these faulty predictions, two recommendations are provided below.
1. Train the model to recognize visual advertisement styles
The model performs relatively well on advertisements and billboards when their surroundings are visible. However, in reality, these surroundings might not always be entirely visible for the camera’s of self-driving cars: billboards are placed on walls right next to roads, while trams with advertisements often narrowly pass cars.
To make the model more robust, it is recommended to not only rely on the surroundings of advertisements and billboards, but also on their visual styles. For instance, the large slogans and often smiling, ecstatic people could provide clues to the model that the advertisements are fiction.
2. Train the model to understand advertisements in motion.
Of course, self-driving cars are usually in motion. This provides an opportunity where the model registers advertisements from afar while their surroundings are still visible. Once the cars get closer and the surroundings disappear, the model could rely on previous detections to understand it is the same advertisements. A combination between this recommendation and the previous one seems ideal.
We love tech, design and culture and get excited about getting AI adopted by a wide variety of audiences.